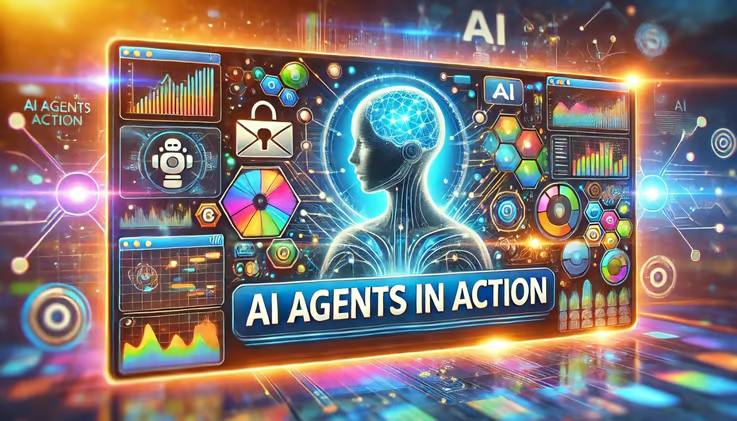
October 11, 2024
5
Why AI Agents Are Leading the Next Generation of Automation
Artificial Intelligence is evolving at an unprecedented rate, and AI agents are at the forefront of this transformation. These agentic applications are designed to autonomously perform tasks, make decisions, and adapt to real-time inputs, allowing them to function with minimal human oversight. As the use of AI agents continues to grow, it’s crucial to explore their development, the hurdles they face, and the profound impact they are set to have on various industries.
What Are Agentic Applications?
Agentic applications are designed to autonomously plan, execute, and adjust tasks based on real-time data and evolving environmental contexts. These systems combine advanced models like large language models (LLMs) and specialized tools to:
- Access diverse data sources: From APIs to real-time financial data, AI agents dynamically integrate information to support decision-making.
- Decompose and streamline tasks: AI agents efficiently break down complex workflows into smaller, manageable steps.
- Adapt and iterate in real-time: AI agents adjust their strategies to achieve better outcomes as new information becomes available.
While AI conversations are often speculative, focusing on prototyping and working examples brings these discussions closer to practical, real-world applications.
The Evolution of AI Agents: A Timeline
Significant milestones in AI research and development have marked the journey toward fully autonomous AI agents:
- 2018: Prompt engineering was introduced to refine natural language processing (NLP) tasks by framing them as question-answer problems, creating a foundational approach for future AI systems.
- 2021: Google researchers introduced the chain-of-thought prompting technique, a breakthrough that enabled AI models to articulate their reasoning step-by-step.
- 2022: OpenAI launched Whisper, an open-source speech recognition model with near-human accuracy.
- 2023: The evolution of Large Language Models (LLMs) expanded to include image, video, and audio processing, followed by the rise of Small Language Models (SLMs) with advanced capabilities in reasoning, dialogue management, and natural language generation.
This progression has resulted in the development of AI agents that are not only capable of complex tasks but can also observe results, iterate on their processes, and deliver improved outcomes dynamically. Their ability to combine thought, action, and observation enables them to tackle intricate problems that mimic human decision-making.
The Emergence of Agentic Applications: Key Examples
As AI agents become more sophisticated, their applications are growing across multiple domains. One prominent example is OpenAgents, a platform designed to deploy agents in real-world scenarios. OpenAgents provides three key types of agents:
- Data Agent: Handles data analysis using Python or SQL, automating routine data manipulation tasks.
- Plugins Agent: Integrates with over 200 APIs to automate scheduling, financial transactions, or communication workflows.
- Web Agent: Enables autonomous web browsing, allowing agents to gather and act on information in real time.
These agents are designed to work within specific environments, providing developers and businesses with powerful tools to build adaptable, autonomous systems capable of solving real-world challenges.
Challenges in AI Agent Development
Despite their enormous potential, AI agents still face several challenges, especially when it comes to user experience and integration:
- User Interface Design: For widespread adoption, AI agents need intuitive, accessible interfaces. Without simplifying the technology, the majority of users—especially non-experts—may struggle to adopt it.
- Seamless API Integration: Agents must operate smoothly across various APIs and data sources to perform complex tasks reliably. Ensuring this seamless interaction can be technically challenging.
- Real-World Adaptability: While agents perform well in controlled settings, real-world environments introduce unpredictable variables that AI systems must be robust enough to handle.
Addressing these challenges will better equip AI agents to provide businesses with reliable, real-time solutions across industries.
The Future of AI Agents: Expanding Capabilities
The future holds exciting developments for AI agents, particularly as they expand into physical environments by integrating robotics and the Internet of Things (IoT). While they currently excel in digital environments—such as web platforms or operating systems like Apple’s Ferrit-UI or Microsoft’s OmniParser—the potential to manage tasks in physical spaces is becoming increasingly real.
Imagine AI agents managing warehouses, supervising manufacturing processes, or assisting healthcare professionals in hospital settings. Microsoft’s recent research on graph-based data architectures paves the way for agents to better understand complex relationships and operate seamlessly in digital and physical environments.
Real-World Examples of Agentic Applications
- Healthcare: AI agents are trained to assist with diagnostic tasks, medical imaging, and even robotic surgery, making healthcare delivery faster and more accurate.
- Manufacturing: In manufacturing, agents help optimize supply chains, manage inventories, and even predict equipment failures by continuously analyzing data.
- Retail: AI agents are becoming crucial in e-commerce, where they streamline processes like customer support, inventory management, and personalized shopping experiences.
- Finance: In the finance sector, AI agents automate fraud detection, trading algorithms, and compliance checks, reducing manual oversight and enhancing decision-making accuracy.
As AI agents evolve, their capabilities will expand into complex environments, providing real-world, measurable benefits across industries.

The rise of AI agents is revolutionizing how tasks are completed, decisions are made, and processes are optimized across industries. From healthcare and manufacturing to finance and beyond, these autonomous systems are ushering in a new era of efficiency and innovation. While the challenges around integration and usability remain, the potential of AI agents to seamlessly operate within both digital and physical environments is vast.
By prioritizing real-world prototyping and focusing on practical applications, businesses can harness the power of AI agents to enhance operations, streamline workflows, and stay ahead in an increasingly competitive landscape.
Subscribe To Get Update Latest Blog Post
Leave Your Comment: