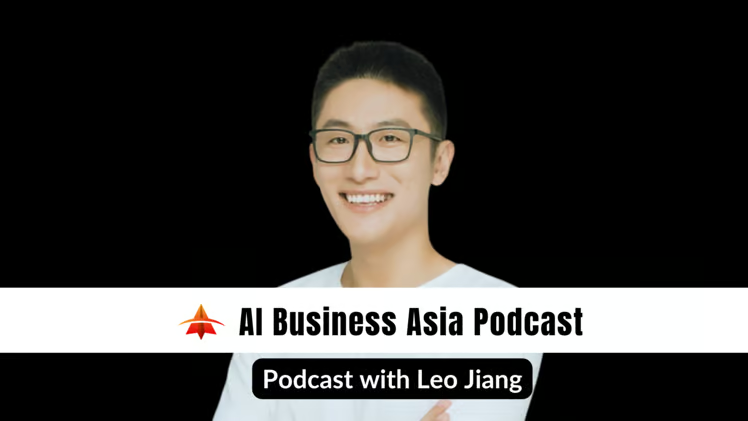
A review on the key points discussed in the 2nd episode of the AI Business Asia Podcast with Lewis Liu, Group Product Manager at Google Gemini and Vertex AI.
If you want to check out the episode → Spotify | Apple
In the fast-paced world of artificial intelligence (AI), particularly in large language models (LLMs), advancements are happening at an unprecedented rate. This recap explores recent developments in Google’s approach to LLMs, including the shift towards incremental launches, the integration of innovative features, and the competitive landscape with global models. We also delve into the role of data, the advantages of first-party data, and what does an enterprise-ready AI experience requires.
Google’s Advancements in LLMs
Over the past year, Google’s team has significantly accelerated the pace of LLM launches, delivering approximately 200 updates compared to the 20-30 launches in previous years, a tenfold increase. This shift reflects a move from traditional, large-scale releases to a more agile, incremental approach.
Innovative Features
- Controlled Generation: Google introduced a controlled generation feature with controlled decoding. This capability allows developers to provide a schema to the model, ensuring that JSON objects generated are 100% accurate and conform to specified schemas. This feature was first delivered with the Gemini 1.5 Pro model and was recently adopted by OpenAI.
- Context Caching: Another innovation is context caching, which helps manage extensive context windows, with Google pioneering a context window of up to 2 million tokens. These advancements highlight Google’s commitment to enhancing model capabilities and user experiences.
Competitive Positioning
Amid perceptions that Google lacks a competitive edge, the company has been focusing on rapid innovation and responding to global advancements in AI. The discussion also touches on the challenges and opportunities presented by emerging models from China, where advancements in long-context handling and fine-tuning for Chinese languages are notable.
The Global Landscape of AI Models
Models from China
Models developed in China are gaining attention for their capabilities, particularly in handling long contexts and tailoring responses to Chinese languages. The conversation reveals that while Google monitors these developments, the company does not directly engage with the Chinese market due to regulatory constraints.
Identity and Nationality in AI
A significant topic of discussion is whether LLMs should have national identities or be influenced by geographic boundaries. The consensus is that while models reflect the biases and identities of their creators, extensive investment in national models may not be necessary. Instead, fine-tuning for specific use cases might be more practical.
The Role of Data in LLM Development
Importance of High-Quality Data
High-quality data remains the cornerstone of training effective LLMs. In the realm of generative AI, the quality and diversity of data directly influence the model’s ability to generate accurate, relevant, and context-aware outputs. Synthetic data, when integrated thoughtfully, can expand the boundaries of what LLMs can achieve, especially in scenarios where real-world data might be limited or biased. The progression of LLMs is increasingly driven by diverse data sources, including human-computer interactions, which enhance the models’ ability to reason, plan, and generate more nuanced responses.
First-Party Data and Infrastructure
- Challenges for Startups: Startups face significant challenges when competing with large enterprises that have access to vast amounts of first-party data. The emphasis on proprietary data and robust infrastructure by companies like Google underscores the critical role these elements play in creating differentiated LLM applications. For startups, the lack of access to first-party data can be a considerable hurdle, making it essential to explore innovative approaches to data acquisition and model training.
- Regulatory Compliance: Grounding AI with factual, reliable information is crucial for the development of enterprise-ready solutions. In the context of generative AI, grounding ensures that the outputs produced by the model are not only accurate but also aligned with the real-world context and regulations. This is particularly important in enterprise applications where the stakes are high, and the cost of inaccuracies can be significant. Adhering to regulatory compliance is another essential aspect, as it ensures that AI solutions operate within the legal frameworks of the industries they serve, reducing the risk of legal complications and fostering trust among users.
Building an Enterprise-Ready AI Experience
Enterprise AI adoption requires careful consideration of several key factors to ensure successful implementation and value creation:
- Platform Capabilities: Enterprises need more than just a model or API. A comprehensive platform should support model fine-tuning, evaluation, and distillation. Collaborative features are crucial, allowing teams to work together on prompts and track changes efficiently.
- Security and Privacy: Robust security measures are paramount. Google, for example, implements strong encryption to ensure customer data remains inaccessible to employees. This level of data protection is critical for maintaining trust and compliance with regulations1.
- Scalability: The ability to deploy models at scale is essential for enterprise applications. This includes considerations for cost-effectiveness and performance optimization.
- Factuality and Grounding: Enterprises require AI systems that provide accurate, factual information. Techniques like Retrieval Augmented Generation (RAG) can help ground AI responses in verified data sources, reducing hallucinations and improving reliability.
- Integration with Existing Systems: For maximum efficiency, AI should seamlessly integrate with an organization’s existing tools and workflows. Google’s approach of incorporating Gemini into widely-used applications like Gmail and Calendar exemplifies this strategy.
- Customization and Fine-tuning: The ability to adapt models to specific industry needs or company data is crucial. This allows enterprises to leverage AI for their unique use cases and proprietary information.
- Compliance and Regulatory Adherence: Enterprise AI solutions must be designed with regulatory requirements in mind, ensuring they meet industry-specific standards and data protection laws.
- Cost Management: While some LLM capabilities are becoming commoditized, enterprises must balance quality, latency, and cost when selecting and implementing AI solutions.
- Continuous Evaluation: Given the rapid pace of AI development, enterprises should implement frameworks for ongoing evaluation of AI models using their own data and use cases, rather than relying solely on public benchmarks.
- Ethical Considerations: Responsible AI development and deployment should be a priority, addressing potential biases and ensuring fair and ethical use of AI technologies
Here are some Google Cloud GenAI Case Studies:
Federal Bank
- Enhanced Chatbot Interaction: Used Vertex AI to make the chatbot, Feddy, more human-like and personalized.
- Multilingual 24/7 Support: Implemented AI for round-the-clock, multilingual customer service.
- API Integration: Developed an API portal to streamline FinTech collaborations and improve security.
- Employee Efficiency: Created a mobile app with Firebase to boost employee productivity.
- Data Security: Used Cloud Armor to protect against DDoS attacks and ensure data compliance.
Mystifly
- Customer Onboarding: Created Mystic, an AI chatbot using Vertex AI, to streamline user onboarding and reduce the need for agent support.
- Enhanced Self-Service: Mystic handles complex queries, improving user satisfaction.
- Scalability: The AI chatbot manages multiple queries at once and escalates unresolved issues to human agents.
CoRover
- BharatGPT Development: Built a multilingual AI platform using Vertex AI, enabling brands to converse with customers in native languages.
- Customization and Security: Offers flexible data usage with Google’s secure infrastructure.
- Scalability: Used Kubernetes Engine’s autoscaling to manage peak traffic efficiently.
- Innovative Collaboration: Partnered with Google Cloud for continuous innovation and customer-focused solutions.
Advice for Startups and Executives
For Startups
Be Nimble: The rapid evolution of AI technology requires startups to remain agile and responsive. Developing a long-term roadmap can be challenging given the unpredictable nature of technological advancements. Startups should focus on quickly adapting to new developments and experimenting with emerging technologies.
For Executives
Evaluate Use Cases: Executives are encouraged to look beyond hype and benchmarks when implementing AI strategies. It is crucial to assess models based on practical use cases and evaluate them with their data. Public benchmarks may not always reflect a model’s performance in real-world scenarios, so hands-on testing is essential.
Conclusion
The landscape of large language models is evolving rapidly, with significant advancements coming from both established players like Google and emerging models globally. Innovations such as controlled generation and context caching are setting new standards for LLM capabilities. As AI technology continues to advance, understanding the role of data, the impact of geographic influences, and the importance of agile development will be key to staying ahead in this dynamic field.
For startups and executives alike, staying nimble and focused on practical applications will be crucial in navigating the future of AI and ensuring successful implementations.
Make sure you’re subscribed to get notifications for our future podcast. episodes → Youtube | Spotify | Apple | RSS
Subscribe To Get Update Latest Blog Post
Leave Your Comment: