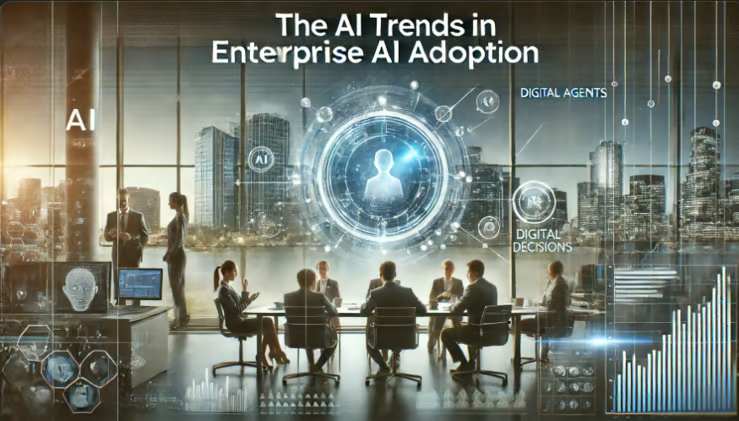

The introduction of ChatGPT marked a watershed moment for enterprises adopting artificial intelligence, highlighting the transformative potential of large language models (LLMs). AI’s ability to generate human-quality text, automate processes, and drive insights has led to rapid adoption across industries.
Fellows Fund, an AI-focused venture capital firm founded by 25 AI experts, closely observes how enterprises are leveraging AI for innovation.
This article will cover:
- The evolution of AI from predictive to generative to agentic capabilities
- The rise of AI-native enterprise applications
- How enterprises are navigating AI challenges in generative AI adoption
Let’s dive in:
AI’s Evolution: From Predictive to Generative to Agentic
AI capabilities have evolved significantly, creating new opportunities for enterprises to harness these technologies. This evolution can be broken down into three major eras:
- Predictive AI Era (1990s-Late 2000s)
Predictive AI was the starting point for many enterprises, focusing on using historical data to forecast future outcomes.
Companies in industries like finance, retail, and healthcare implemented predictive algorithms to optimize operations, from demand forecasting to risk assessment. This era marked AI’s utility in making informed business decisions based on data-driven insights.
- Generative AI Era (2010s-Present)
The current era of generative AI has transformed the landscape for enterprises. With advanced models like OpenAI’s GPT-3, AI can now generate new content, automate creative tasks, and enhance customer engagement.
This era is driving significant innovation in content creation, marketing, customer service, and more. Generative AI also enables enterprises to scale up personalization efforts, improve productivity, and create new revenue streams through automated processes.
Generative AI is often likened to technological breakthroughs such as the internet and cloud computing. Its ability to process vast datasets and produce outputs at scale has made it a cornerstone for many enterprise applications. From personalized marketing campaigns to AI-driven content generation, enterprises are leveraging AI to enhance efficiency and user engagement.
- Agentic AI Era (Emerging)
The era of agentic AI is on the horizon, bringing autonomous agents capable of executing complex tasks with minimal human input.
Enterprises will increasingly rely on agentic AI to automate operations, orchestrate workflows, and manage both digital and physical processes. This next evolution will transform how businesses function, driving productivity and enabling seamless decision-making in real-time environments.
As agentic AI matures, enterprises will face challenges in balancing automation with ethical considerations, transparency, and control. But those who can successfully integrate autonomous agents will see significant gains in efficiency and scalability.
The Rise of AI-Native Enterprise Applications
Many enterprises are transitioning from AI-enhanced products to AI-native applications—tools and systems built from the ground up with AI as their foundation. This shift is fundamentally changing how enterprises approach problem-solving and service delivery.
Unlike traditional enterprise software, where AI features are added as enhancements, AI-native applications embed AI at every level. These applications are designed to adapt dynamically to user needs, learn continuously, and deliver more personalized and intelligent services.
For instance, Microsoft’s Copilot integrated into Office 365 allows employees to use natural language prompts to generate documents, presentations, and analysis. in my recent interview with Microsoft champaign – Adeel Khan
#5 Teaser Microsoft AI Co-Pilot : How to power Enterprise AI Transformation with Microsoft Copilot?
Highlights of the podcast episode:
- Co-Pilot represents a major shift in organizational operations.
- It democratizes AI capabilities for managers and employees.
- Personal experiences with Co-Pilot highlight its practical benefits.
- Co-Pilot acts as a smart assistant, enhancing productivity.
- Healthcare professionals benefit from Co-Pilot’s ability to streamline documentation.
Similarly, Gamma and Opus Clip, AI-native platforms for presentations and video editing, offer enterprises greater efficiency in creative processes through automated workflows. Enterprises are now able to cut time-consuming tasks while improving output quality, allowing their teams to focus on strategic goals.
AI-native applications are not just limited to operational improvements—they are also transforming customer experiences. AI-driven customer support platforms, for example, can respond to customer inquiries with more precision and personalization, creating more seamless user experiences. As enterprises integrate these AI-native solutions, they unlock new possibilities in workflow optimization and service innovation.
Navigating AI Challenges in Generative AI Adoption
Despite the impressive capabilities of generative AI, enterprises face several key challenges as they seek to harness its full potential:
- Accuracy and Reliability
Generative AI models are known for occasional inaccuracies, or “hallucinations,” where the AI generates plausible but incorrect information. For enterprises, especially those in regulated industries like finance or healthcare, ensuring accuracy is crucial. These sectors require AI outputs to be consistent and reliable, and reducing the incidence of hallucinations will be a major focus for AI providers. - Data Management and Security
Data is the lifeblood of any AI system, and managing it effectively is one of the biggest hurdles for enterprises. Ensuring that data is high-quality, secure, and compliant with regulations like GDPR or HIPAA is essential for successful AI adoption. Data privacy and security are also critical concerns, as AI systems often process sensitive information that requires careful handling. - Complexity of Integration
AI systems are not standalone solutions—they need to integrate seamlessly into existing enterprise IT infrastructures. This requires careful planning, customization, and scalability to ensure that AI systems can operate effectively within complex enterprise ecosystems. Enterprises must invest in the right tools and platforms to ensure that AI applications are adaptable and can evolve with business needs. - Return on Investment (ROI)
With AI investments growing, enterprises are under pressure to demonstrate measurable ROI from their AI initiatives. This can be particularly challenging with generative AI, where the benefits are often spread across different functions such as automation, customer engagement, and creative content generation. Enterprises need to develop robust frameworks for measuring AI’s impact on efficiency, cost savings, and revenue growth.

- AI is evolving from predictive to generative and now agentic, offering enterprises new tools for automating processes and driving innovation.
- AI-native applications are transforming how enterprises operate, allowing for greater adaptability, efficiency, and personalization.
- Enterprises must address key AI challenges—such as accuracy, data management, and ROI—if they want to fully capitalize on the potential of generative AI.
- As AI continues to evolve, enterprises that can effectively navigate the trends and AI challenges will be best positioned to lead in the next era of business innovation.
Subscribe To Get Update Latest Blog Post
Leave Your Comment: